Smart sensors can teach themselves AI models.
Smart Sensors Teach AI – A new technique enables on-device training of machine-learning models on edge devices like microcontrollers in sensors and IoT devices, which have very limited memory. This could allow edge devices to continually learn from new data, eliminating data privacy issues, while enabling user customization, and enhancing AI capabilities of security and automation systems.
Microcontrollers, miniature computers that can run simple commands, are the basis for billions of IoT connected devices, including sensors. But cheap, low-power microcontrollers have extremely limited memory and no operating system, making it challenging to train artificial intelligence models that work independently from central computing resources.
Training a machine-learning model on an intelligent edge device allows it to adapt to new data and make better predictions. However, the training process requires so much memory that it is typically done using powerful computers at a data centre, before the model is deployed on a device. This is more costly and raises privacy issues, since user data must be sent to a central server.
To address this problem, researchers at MIT and the MIT-IBM Watson AI Lab developed a new technique that lets smart sensors teach AI to themselves via on-device training using less than a quarter of a megabyte of memory. Other training solutions designed for connected devices can use more than 500 megabytes of memory, greatly exceeding the 256-kilobyte capacity of most microcontrollers.
The intelligent algorithms and framework the researchers developed reduce the amount of computation required to train a model, which makes the process faster and more memory efficient. Their technique can be used to train a machine-learning model on a microcontroller in a matter of minutes.
This technique also preserves privacy by keeping data on the device, which could be especially beneficial when data are sensitive, such as in medical applications. It also could enable customization of a model based on the needs of users. Moreover, the framework preserves or improves the accuracy of the model when compared to other training approaches.
“Our study enables IoT devices to not only perform inference but also continuously update the AI models to newly collected data, paving the way for lifelong on-device learning,” said Song Han, an associate professor in the Department of Electrical Engineering and Computer Science (EECS), a member of the MIT-IBM Watson AI Lab, and senior author of the paper.
“The low resource utilization makes deep learning more accessible and can have a broader reach, especially for low-power edge devices.”
A common type of machine-learning model is known as a neural network. Loosely based on the human brain, these models contain layers of interconnected nodes, or neurons, that process data to complete a task, such as recognizing people in photos. The model must be trained first, which involves showing it millions of examples so it can learn the task. As it learns, the model increases or decreases the strength of the connections between neurons, which are known as weights.
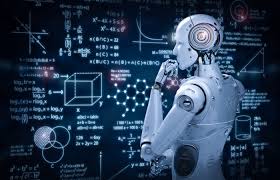
The model may undergo hundreds of updates as it learns, and the intermediate activations must be stored during each round. In a neural network, activation is the middle layer’s intermediate results. Because there may be millions of weights and activations, training a model requires much more memory than running a pre-trained model, Han explains.
Han and his collaborators employed 2 algorithmic solutions to make the training process more efficient and less memory-intensive. The first, known as sparse update, uses an algorithm that identifies the most important weights to update at each round of training. The algorithm starts freezing the weights one at a time until it sees the accuracy dip to a set threshold, then it stops. The remaining weights are updated, while the activations corresponding to the frozen weights don’t need to be stored in memory.
“Updating the whole model is very expensive because there are a lot of activations, so people tend to update only the last layer, but as you can imagine, this hurts the accuracy. For our method, we selectively update those important weights and make sure the accuracy is fully preserved,” Han said.
Their second solution involves quantized training and simplifying the weights, which are typically 32 bits. An algorithm rounds the weights, so they are only 8 bits, through a process known as quantization, which cuts the amount of memory for both training and inference. Inference is the process of applying a model to a dataset and generating a prediction. Then the algorithm applies a technique called quantization-aware scaling (QAS) to help smart sensors teach AI, which acts like a multiplier to adjust the ratio between weight and gradient, to avoid any drop in accuracy that may come from quantized training.
Smart Sensors Teach AI
The researchers developed a system, called a tiny training engine, that can run these algorithmic innovations on a simple microcontroller that lacks an operating system. This system changes the order of steps in the training process, so more work is completed in the compilation stage, before the model is deployed on the edge device.
“We push a lot of the computation, such as auto-differentiation and graph optimization, to compile time,” Han explained. “We also aggressively prune the redundant operators to support sparse updates. Once at runtime, we have much less workload to do on the device.”
Their optimization only required 157 kilobytes of memory to train a machine-learning model on a microcontroller, whereas other techniques designed for lightweight training would still need between 300 and 600 megabytes.
They tested their framework by training a computer vision model to detect people in images. After only 10 minutes of training, it learned to complete the task successfully. Their method was able to train a model more than 20 times faster than other approaches.
Now that they have demonstrated the success of these techniques for computer vision models, the researchers want to apply them to language models and different types of data, such as time-series data. At the same time, they want to use what they’ve learned to shrink the size of larger models without sacrificing accuracy, which could help reduce the carbon footprint of training large-scale machine-learning models.
This smart sensors teach AI work is funded by the National Science Foundation, the MIT-IBM Watson AI Lab, the MIT AI Hardware Program, Amazon, Intel, Qualcomm, Ford Motor Company, and Google.
More news from SEN.
“Smart Sensors Teach AI”